

6608–6617).Įlhayek, A., de Aguiar, E., Jain, A., Tompson, J., Pishchulin, L., Andriluka, M., Bregler, C., Schiele, B., & Theobalt, C. In Proceedings of the IEEE/CVF conference on computer vision and pattern recognition (pp. Hope-net: A graph-based model for hand-object pose estimation. arXiv preprint arXiv:1908.10357.ĭoosti, B., Naha, S., Mirbagheri, M., & Crandall, D.
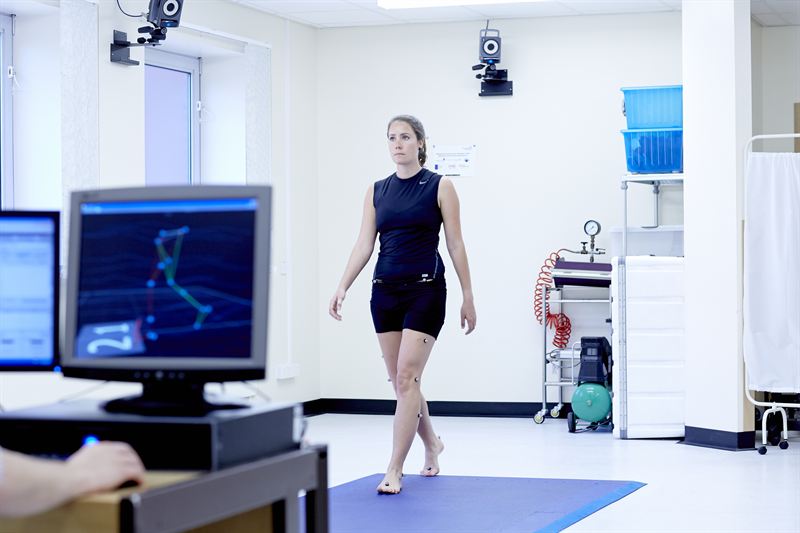
Bottom-up higher-resolution networks for multi-person pose estimation. Human4d: A human-centric multimodal dataset for motions and immersive media. Sensors, 19(2), 282.Ĭhatzitofis, A., Saroglou, L., Boutis, P., Drakoulis, P., Zioulis, N., Subramanyam, S., Kevelham, B., Charbonnier, C., Cesar, P., Zarpalas, D., et al. Deepmocap: Deep optical motion capture using multiple depth sensors and retro-reflectors. In Proceedings of the IEEE conference on computer vision and pattern recognition (pp. Realtime multi-person 2d pose estimation using part affinity fields. 3D pictorial structures for multiple view articulated pose estimation. Springer.īurenius, M., Sullivan, J., & Carlsson, S. In Iberian conference on pattern recognition and image analysis (pp. Evaluating the impact of color information in deep neural networks. View invariant human body detection and pose estimation from multiple depth sensors. thesis, Universidad del País Vasco-Euskal Herriko Unibertsitatea.īekhtaoui, W., Sa, R., Teixeira, B., Singh, V., Kirchberg, K., Yj, Chang, & Kapoor, A. Cloud point labelling in optical motion capture systems. Computers & Graphics, 69, 59–67.īascones, J. Real-time labeling of non-rigid motion capture marker sets.
#Vicon motion capture professional
DeMoCap is driven by a special dataset captured with 4 spatio-temporally aligned low-cost Intel RealSense D415 sensors and a 24 MXT40S camera professional MoCap system, used as input and ground truth, respectively.Īlexanderson, S., O’Sullivan, C., & Beskow, J. We simultaneously handle depth and marker detection noise, label and localize the markers, and estimate the 3D pose by introducing a novel spatial 3D coordinate regression technique under a multi-view rendering and supervision concept. We introduce an end-to-end differentiable markers-to-pose model to solve a set of challenges such as under-constrained position estimates, noisy input data and spatial configuration invariance. Trading off some of their typical features, our approach is the sole robust option for far lower-cost marker-based MoCap than high-end solutions. We introduce DeMoCap, the first data-driven approach for end-to-end marker-based MoCap, using only a sparse setup of spatio-temporally aligned, consumer-grade infrared-depth cameras. Optical marker-based motion capture (MoCap) remains the predominant way to acquire high-fidelity articulated body motions.
